Insurers and intermediaries digitize their companies more and faster. This has implications for the organizational functions that support this such as the Data Science and AI teams. From our recently conducted Data Science Maturity Quick scan among Dutch Insurers we learn that nearly all companies currently organize their Data Science in the same way, centrally. However, the front runners are now about to transition to a different, hybrid, organizational model. Determining the best organizational model for the Data Science function turns out not to be simple.
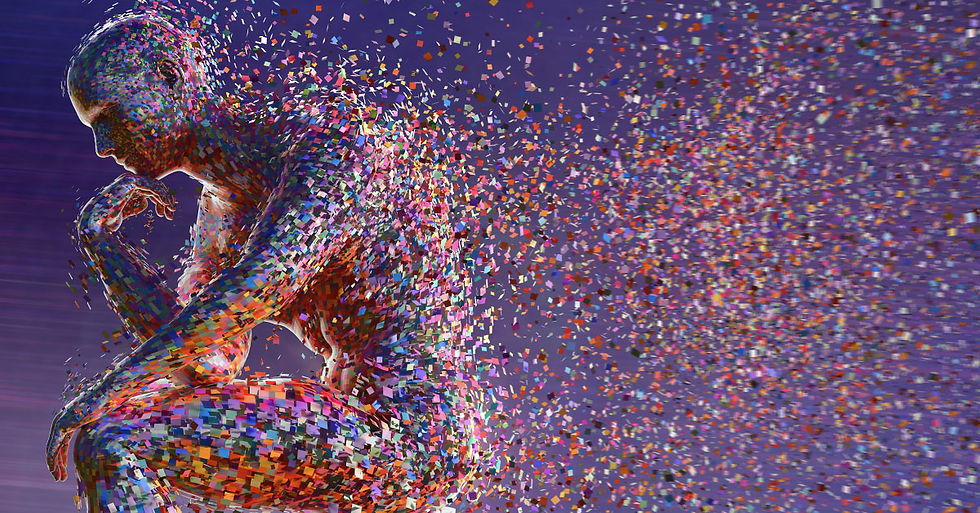
How do you organize Data Science and AI as a scalable corporate function, when you can no longer keep it centrally organized and also do not want to switch to a very decentralized, hybrid model?
Three basic models Actuary expertise and business intelligence have been part of the insurance business for a long time. But since about two decades people with the title Data Scientist started to appear. These professionals usually worked on non-risk use cases, such as in Sales, Marketing & Distribution, Fraud and Customer Service and they wanted to apply the latest, often non-linear, Machine Learning techniques (ML). The introduction of this kind of work often happened very decentralized and scattered throughout the organizations. But as the reputation and expectations of their work grew, these new professionals were often grouped together in central Centers of Competence (CoC).
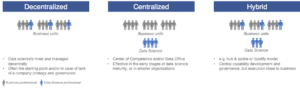
Fig 1. Three basic models for organizational functions
The CoC model brings some advantages for a new data science function, when compared to the decentralized model. Especially when the company is not yet really functioning like a digital, data driven organization. Five out of six organization in our Maturity Quick Scan have organized their data scientists in a CoC model. However, at some companies, the digital transformation is getting serious and, in that case, strong centralization can result in a capacity bottleneck. Or be the cause of too big of a gap between business and data sience teams in terms of knowledge, priorities and communication.
Switching from a centrally organized model to a more hybrid model is often advised as a best-of-both-worlds solution. This should make it easier to scale up and align knowledge and application of data science closely with the day to day business, while a select number of activities and governance can remain central.
Concerns over hybrid models Spotify developed a popular hybrid version that has become known as the Spotify model with its Squads, Tribes, Chapters and guilds1. This type of model has become popular among the digital natives and e-commerce companies in this world. But many data science managers in Dutch insurance companies have their concerns about this highly decentralized version of a hybrid model. These concerns are;
Data culture and scale of the AI applications may be insufficient still to maintain the needed continuous development and innovation
There are often still company-wide use cases yet to be developed that could be realized much more efficiently centrally
Next to that, IG&H has identified there is a large gap in data science maturity between corporate insurance and consumer insurance2. A more central organization model can help to narrow that gap
This is why in practice we often choose for a more central version of the hybrid model. But every hybrid (re)organization raises questions such as;
How do you keep the complexity of funding and governance down?
How do you maintain sufficient control over standards, continuous development and innovation?
Which activities and responsibilities do you keep central and which not?
A different perspective; a successful retail business model Thinking about these questions from a different perspective or from the perspective of a different sector can help to find answers. We do this a lot to solve problems at IG&H. In this article we make an analogy with a good ol’, trusted, business model. In the late fifties of the 20th century fast food giant McDonalds started to conquer the world in rapid pace. The McDonalds concept (branding and methodology) was a proven success, but the explosive, profitable growth was partly made possible by the chosen business model for expansion, the franchise model.
A franchise is a type of business that is operated by an individual(s) known as a franchisee using the trademark, branding and business model of a franchisor. In this business model, there is a legal and commercial relationship between the owner of the company (the franchisor) and the individual (the franchisee). In other words, the franchisee is licensed to use the franchisor’s trade name and operating systems.
In exchange for the rights to use the franchisor’s business model — to sell the product or service and be provided with training, support and operational instructions — the franchisee pays a franchisee fee (known as a royalty) to the franchisor. The franchisee must also sign a contract (franchise agreement) agreeing to operate in accordance with the terms specified in the contract.
A franchise essentially acts as an individual branch of the franchise company.
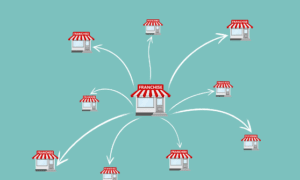
Fig 2. Illustration of the franchise model Credits: https://franchisebusinessreview.com/post/franchise-business-model/
The franchise model offers some important benefits to a business concept that help to expand fast and durable;
Access to (local) capital
Entrepreneurship of local establishments
Presence close to local customers
Ability to facilitate centrally what locally cannot be realized in a cost-efficient way, or what is an essential part of the success formula (e.g. R&D, Production, Procurement and Marketing)
Each of these qualities can be related to lessons learned and best practices of scaling up Data Science within an organization. As the franchise principle made it possible for McDonalds to rapidly expand into the world, it can also help expand Data Science throughout the company.
Applying franchise model principles Many insurers desire a more adaptable and entrepreneurial company culture. Ownership, client focus and flexibility are key words. The principle of local entrepreneurship in the franchise model fits very well with this. It encourages both the business responsible as well as the data scientist to work very client-focused and always with a business case to achieve.
Data Science is a broad discipline and it rapidly evolves while it is often not yet embedded well in most companies. This makes a central organizational element very valuable to ensure quality standards, continuous development and innovation. Just like the franchiser in the franchise model often facilitates certain means of production, R&D and best practices. One of the front runners in the Dutch insurance market with respect to organizing their data science is therefore transitioning to franchise-inspired organization model for their data science function.
So, franchise-inspired thinking can be valuable for Data Science organizations that want to move beyond the CoC, but not (yet) want to move to a very decentralized hybrid model like the Spotify model. However, which organizational model and specific choices are the best fit is naturally dependent on multiple variables. Among others; company structure and company culture, the digital maturity of the organization and the maturity of the data science function itself. Because the value of data science and AI are completely dependent on the quality of collaboration with the business and on broad application throughout the company it is vital to objectively assess the current situation and to strike the right balance between centralized and decentralized, with a company-specific touch.
Contact Mando Rotman E: mando.rotman@igh.com Manager Data Science IG&H
Jan-Pieter van der Helm E: janpieter.vanderhelm@igh.com Director Financial Services IG&H
1) https://agilescrumgroup.nl/spotify-model/ 2) https://www.igh.com/news/page/2/ 3) https://franchisebusinessreview.com/post/franchise-business-model/
Comments